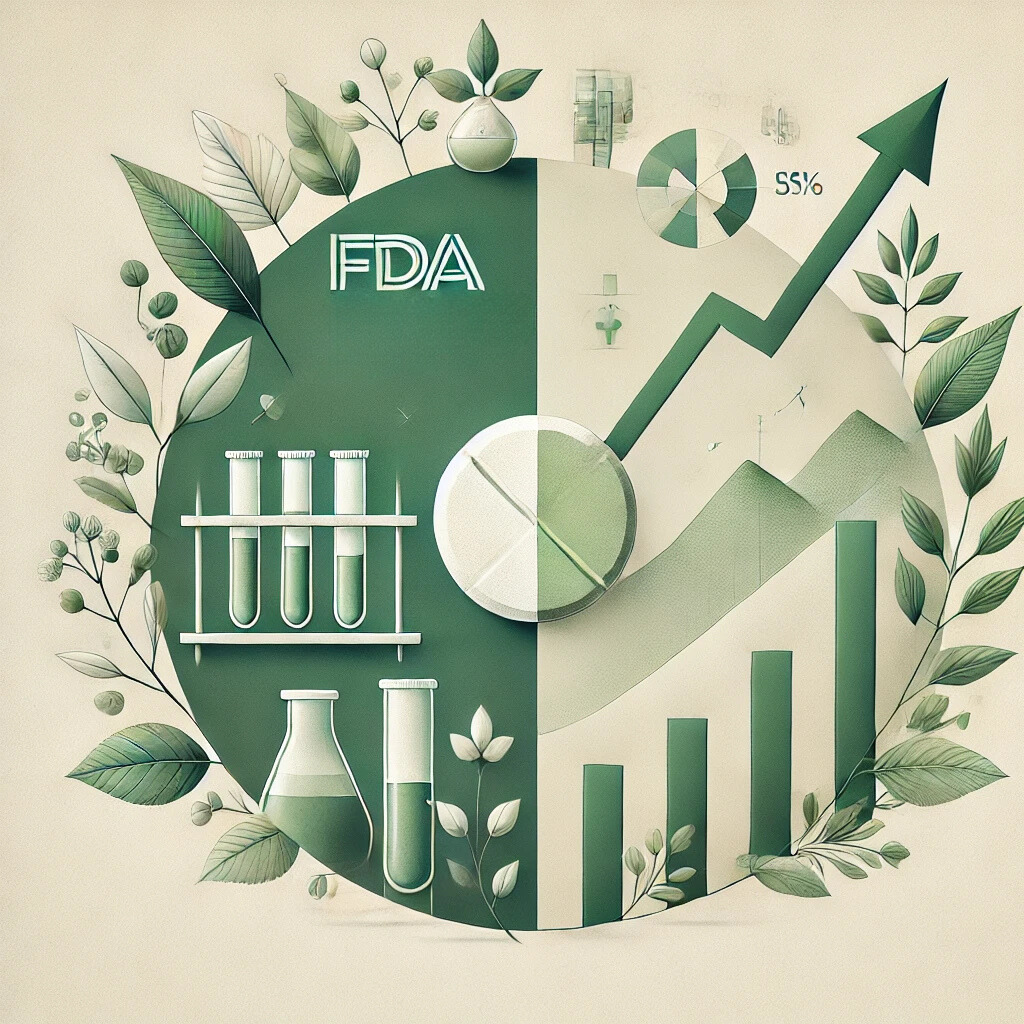
The Upside of Uncertainty
It is human nature to seek explanations, especially when faced with our collective anxieties and uncertainties. Yet, we often turn a blind eye to the explanations already provided by scientists and experts, choosing instead to ignore well-established facts. Consider our response to the climate crisis—despite clear evidence of our growing environmental impact on climate change, many still dismiss or downplay the scientific consensus. At the same time, we are drawn to captivating stories and dreams, leading us to embrace irrational optimism and inflate market valuations beyond reason.
Should we be concerned about a possible collapse of certain major currencies? Given the horrific fighting going on in Eastern Europe, the Middle East, and elsewhere… should we be worried about possible catastrophic events that change our future? How will these events affect our financial markets? If things are about to get wild, should we just liquidate our positions and wait for the geopolitical perturbations to subside?
Why do stocks go up?
“Stocks are going up only because companies are purchasing their own shares,” says a financial market commentator. Why would a company want to purchase its own shares?
Corporate finance theory says that it should do so when a company thinks its stock is undervalued and has cash surpluses, assuming investors are happy with the company buying its own stock rather than paying dividends.
Some argue that companies should buy their own stock “when they know better than anyone what is happening at their company.” This is, of course, a funny argument since they should know what is happening at their company better than anyone else. Otherwise, why should they be given a mandate to manage the company?
What other explanations do pundits usually give for stocks that continue to soar despite growing scepticism about the company’s growth prospects, with many believing they are significantly exaggerated amid a landscape filled with risks and uncertainties? We often hear the following quotes from financial commentators about why certain stock prices go up: “The Fed is getting ready to cut rates,” or “Funding packages from Congress are pumping money into the economy.” Although generally impactful, such macro-economic factors usually have little to do with why individual stocks go up or down.
In the spirit of Occam’s razor, the simplest explanation may be the best:
After supply constraints due to the pandemic shutdowns, commodity and utility costs have sunk down, and demand for consumer products has picked up. So, costs are lower, revenues are higher, and profits are up. As a result, manufacturers of goods whose operating costs are sensitive to such factors can effectively produce again. Here is a simple explanation why the stock price of this manufacturer may be up!
Or, consider a macro-economic explanation:
The stock market indices are going up because companies are generating better profits through improved efficiencies compared to the past five years. Inflation and unemployment remain in check, and households are recently more confident in allocating a larger portion of their wealth to the stock markets.
When businesses thrive and the public accounts are balanced, their excess funds can be reinvested for further growth. Simpler explanations are easier to understand and approve. This helps create a bullish sentiment which can propel the markets forward.
What about Artificial Intelligence?
AI is boosting productivity across industries. This seems like a simple explanation. But such performance depends on the context. For instance, let’s take the case of the pharmaceuticals industry. AI increases the speed at which biotech firms discover new molecules to create more effective new drugs. But how long does it really take to develop a drug, get it approved by the FDA, and manufacture it in sufficient amounts to bring it to the markets? Can AI make this process better?
The Long Game of Drug Development
Imagine you’re trying to find your way through a vast maze, but there’s no map and no one to guide you. You wander through the twists and turns, hoping to stumble upon the exit. Every wrong turn costs you precious time, and if you get lost too many times, you might have to give up. You finally reach a promising exit, but you still need to pass a panel of gatekeepers. That’s drug development. When you find the exit, the FDA will assess all the choices you made along the way. If they find any missteps, you’ll have to retrace your steps and navigate the maze again. Only a few escape the maze, while many others remain trapped in the labyrinth of uncertainty.[1]
The drug development process starts from the moment you have a formula for a new molecule, and it typically takes 10 to 15 years to see through with the drug ending in your medicine cabinet. The first leg of the process is discovery. At this pre-trial stage, your researchers screen thousands of compounds, hoping that one does something interesting. This is your first real expenditure of time and resources, taking anywhere from 3 to 6 years. Let’s say you find something. You must now file your first “Investigational New Drug” (IND) application with the FDA. If the FDA approves, you’re off to the clinical trials.
In the Phase 1 trials, you will test your drug on a small group of healthy volunteers—just enough to make sure your miracle cure doesn’t harm anyone outright. These Phase 1 trials take between 6 and 8 years. If your drug survives this first phase, you can now spend another 1 to 2 years testing on hundreds of actual patients who might benefit. About one-third of drugs pass Phase 2.
Then, you are off to the big leagues. Now, you will be testing on thousands of patients, often across multiple countries, trying to prove your drug not only works but works better than everything else. The estimated direct daily cost to conduct a clinical trial is approximately $40,000 per day for phase II and III clinical trials.[2] This will typically take 3 to 4 years. After this, there’s still one last hurdle: the New Drug Application (NDA). This is the pitch meeting where you convince the FDA that your drug is ready for the world.
Now, the FDA will take about 6 to 10 months to review your data, potentially longer if they request more trials, more evidence, or just more time to sift through the hundreds of thousands of pages of clinical results you’ve provided them. If all goes well, the FDA will give you their stamp of approval. Now, you’ll have to manufacture the drug. You’ll need specialized factories to mass produce the drug. Building a manufacturing plant may take a few more years and tens of millions of dollars, depending on your drug’s complexity. Only then can the drug hit the shelves. Well, sort of. After that, there’s marketing, pricing negotiations, insurance coverage debates, and a whole lot of PR to make sure your drug actually gets into patients’ hands.
From the lab to your medicine cabinet, we are talking about a billion-dollar bet, a 10 to 15 years time horizon, and a less than 10% survival rate. But when it works, it is all worthed: when the drug starts changing lives, it feels like a miracle.
How much do AI-aided discoveries account for the performance of the biotech industry?
Enter AI, your shiny maze whisperer, promising to transform the daunting labyrinth into a more manageable journey by offering insights. In drug discovery, AI is playing the role of a supercharged assistant. Instead of chemists staring at Petri dishes, you’ve now got algorithms crunching through billions of molecular combinations. And this is happening in hours, not years. Finding the right molecule that might latch onto that problematic protein is now getting pinpointed much faster, shortening the first leg of the drug development process. AI models predict how a drug might behave before it is even tested in a real tube. Thus, AI promises to map biological pathways faster than was possible before.
Clinical trials, the notorious time-and-money sink, are getting a tech boost, too. Instead of the old-fashioned way of recruiting patients—slow, manual, and rife with delays—AI is helping identify the ideal trial participants in a fraction of the time. It’s optimizing trial designs and predicting adverse effects. Data can now be analyzed faster, with AI spotting trends humans might miss or take longer to identify. Meanwhile, AI may also be shaving some time off the clinical phase for certain drugs.
Manufacturing may also be streamlined. AI models may be able to simulate how large-scale production may unfold, predicting issues before they arise. They can help optimize the supply chain, making sure production ramps up efficiently.
In theory, the AI enthusiasts argue, the technology could take what was once a 10- to 15-year process and cut it down to 7 years. This AI narrative, however, may be overlooking serious challenges such as the complexity of biological systems, data quality issues, and the risk of algorithmic biases. While AI can accelerate the identification of promising compounds, it relies heavily on existing data, which may be incomplete or flawed, leading to misleading predictions. Additionally, the nuanced nature of human biology means that an AI model could overlook critical interactions and safety concerns, potentially resulting in costly failures in clinical trials. Without careful oversight and validation, the promise of AI could fall short, leading to disillusionment in the drug development process.
Although AI will some day streamline operations everywhere, making businesses more efficient and profitable, the question of how much value AI accounts for is far less obvious than it seems at first consideration. According to one survey, employees in the pharmaceutical industry are seen sceptical about the effectiveness of AI technologies; despite the hype surrounding generative AI, most respondents considered it overrated.
At the moment, three companies are viewed as the pioneers leading the charge in AI:
The first two companies, Microsoft provides cloud computing resources and OpenAI advances cutting-edge models. But it is Nvidia which seems to lead the pack as it delivers the high-performance GPUs essential for AI computations. Together, their platforms and tools form the core ecosystem for businesses to create, deploy, and scale AI solutions.
Like the engineers of the digital economy, these three companies are building the essential components that make innovation possible, leaving the broader market to take these tools to new heights.
Nevertheless, AI will most likely become the new driver of the global economy only when thousands of companies each prove how they can build better products, cut costs, increase profits, and innovate better with their applications.
In Conclusion
Rate cuts or share buybacks can push markets higher, but the core reason stocks are rising is that companies are performing better, fueled by technology that is solving age-old problems and driving faster-than-expected profits.
There is an old saying among traders that It’s all about “controlling fear and greed.”
You just need to implement some smart strategies to navigate the short-term downside risks of your key positions while making sure that your portfolios maintain adequate exposure to the potential upside that are provided by sound investment opportunities.
[1] https://www.norstella.com/why-clinical-development-success-rates-falling/
[2] See: National Center for Biotechnology Information, pubmed.ncbi.nlm.nih.gov/38773058/
Post a Comment
You must be logged in to post a comment.